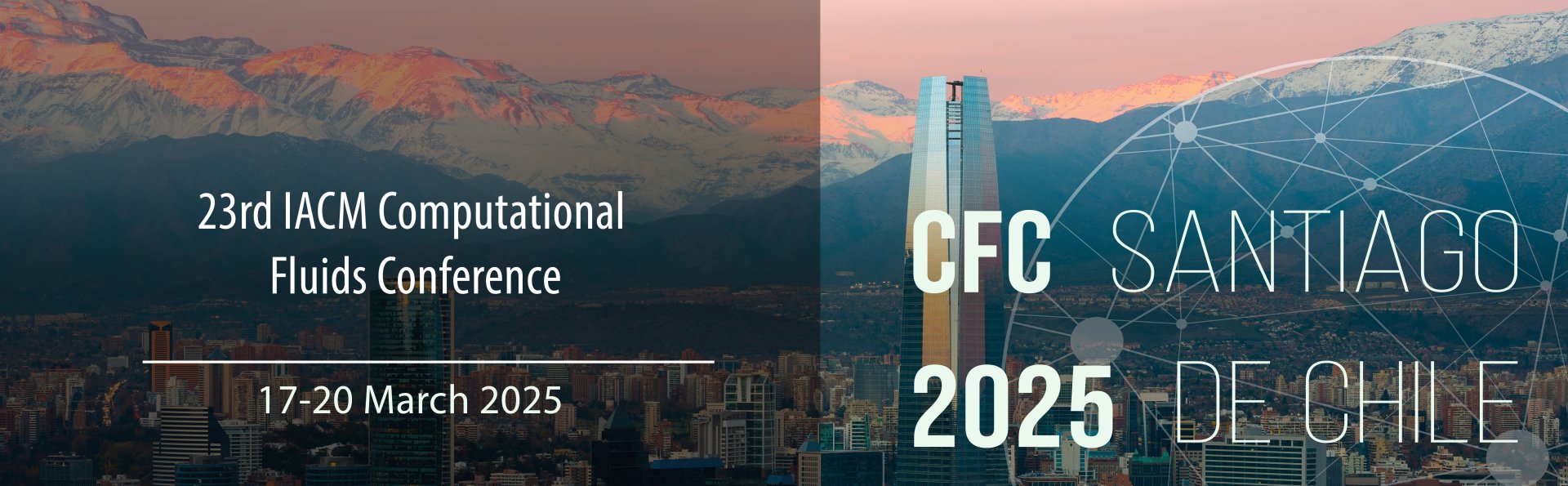
MS008 - Data-Driven Methods for Heat Transfer and Fluid Flow
Keywords: data-driven methods, Deep Learning Discretizations, digital twins, Scientific ML
With advancement in Artificial Intelligence, machine learning and inverse modelling methods, data-driven approaches have become increasingly popular for predicting heat and fluid flow behaviour in complex physical systems. The goal is to transfer knowledge learnt from training data on a particular scenario and apply it to new situations which the model had not seen before. Three prominent data-driven methods for fluid flow modelling are Physics-Informed Neural Networks (PINNs), Graph Network Simulators (GNSs) and Convolutional Neural Networks (CNNs).
All three approaches have shown great potential for accurately simulating heat transfer and fluid flow phenomena. These interactions between different parts of the fluid system, such as turbulent flows or multi-phase flows can be dynamically learned by the model - and produce accurate inference results. However, there are still challenges to be overcome in using these models from generalisation ability to accurately capture complex fluid flow phenomena such as free surface flows and fluid splashing. The GNS models have shown promise in areas such as particle motion data and free surface flow capture however, they require large amount of training data to achieve accurate predictions. PINNs are limited by the quality and quantity of the data used for training and may not generalise well to new or complex situations. CNNs predict fluid flow by visualising it as a time series of images rather than learning physical laws that govern particle interaction.
The mini symposium aims to bring together authors to stimulate discussion on using deep learning models including, and not limited to, the following three topics:
1. Novel deep learning models for heat and fluid flow that can capture complex phenomena such as free surface flows, multi-phase flows, fluid-structure interactions, and turbulent flows.
2. Hybrid approaches that combine physics-based models with data-driven methods to enhance the accuracy and efficiency of simulations.
3. The generalisation ability of deep learning models on Out-of-Distribution (ODD) examples.