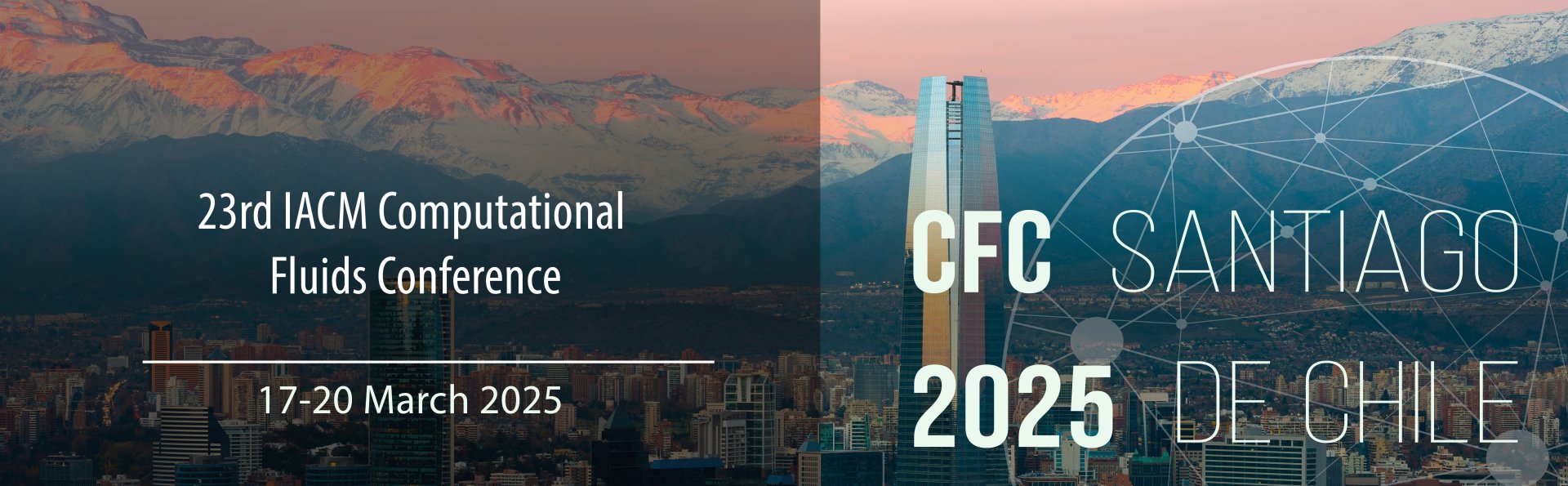
Cause-and-effect approach to reduced-order modeling of turbulence
Please login to view abstract download link
Advancing our understanding of turbulent flows requires the development of low-order representations capable of capturing their high-dimensional, chaotic, and multi-scale nature. Here, we employ a causal inference method to inform the development of reduced-order models tailored to the dynamics of turbulent flows. The method, referred to as SURD (Synergistic-Unique-Redundant Decomposition), assesses causality by quantifying the increments of information obtained about future events based on combinations of past events. The causal interactions among these events are further decomposed into redundant, unique, and synergistic contributions according to their nature. Quantifying these causal contributions from a set of input variables to a target variable facilitates the selection of minimal yet effective sets, which aids the construction of models and enhances their interpretability. In this work, we demonstrate the application of SURD for reduced-order modeling to predict the wall shear stress in a turbulent boundary layer using turbulent velocity signals. We show how SURD-guided feature selection enables the construction of efficient and accurate low-order models by identifying the most relevant input variables. In synergistic cases, where multiple variables collectively influence the target, incorporating all synergistically-interacting inputs improves significantly prediction accuracy. Conversely, in redundant cases, where variables contribute interchangeable information to the target, the model can be optimized by selecting the most practical variable, which reduces data requirements without compromising performance.