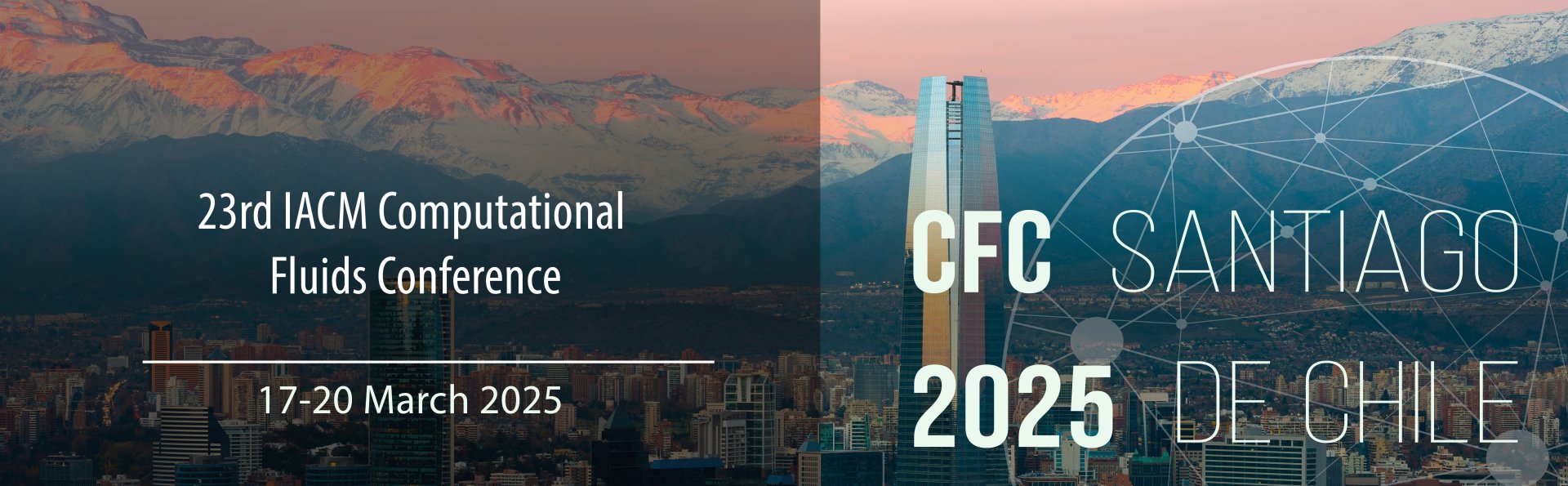
Data-Driven Reduced Order Modeling of Extreme Events in Turbulent Flows
Please login to view abstract download link
Extreme and rare events are common features of many chaotic dynamical systems found in nature, such as earthquakes, solar coronal mass ejections, and rapid fluctuations in turbulent flows. While the events may be rare, they can pose a threat to safe and resilient operations; therefore it is critical to model, forecast, and even mitigate these events if possible. In this work, we obtain a data-driven set of linear projections using Covariance Balancing Reduction using Adjoint Snapshots (CoBRAS) to identify a meaningful set of low-dimensional coordinates for modeling the energy dissipation of the chaotic Kolmogorov flow. We demonstrate the usefulness of these coordinates by using them to design a simple feedback controller that prevents extreme energy dissipation events. We compare the data-driven approach to past analytic approaches developed from theory and discuss the application to other engineering systems.