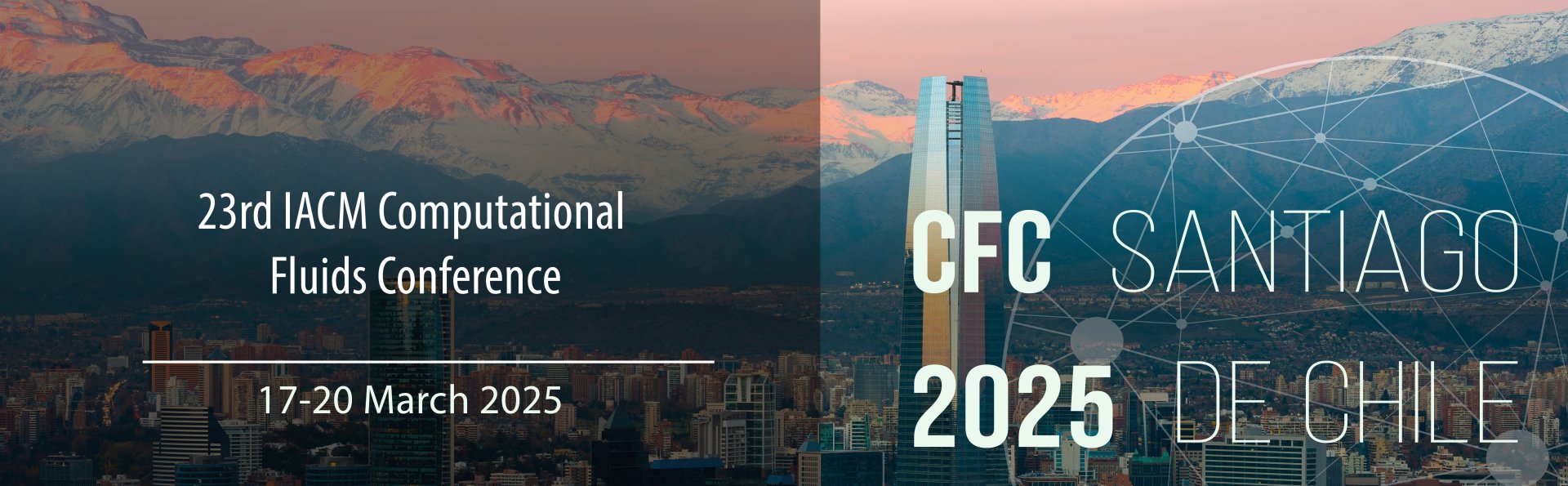
Mesh-Free Pressure Field Reconstruction from Image Velocimetry Data Using SIREN
Please login to view abstract download link
Recent advancements in image-based experimental techniques, such as particle image velocimetry (PIV) and Lagrangian particle tracking (LPT), have enabled high-resolution velocity measurements, facilitating non-intrusive pressure field reconstruction. Traditional methods like omni-directional integration (ODI) and Green's function integral (GFI) are mesh-based and face challenges with unstructured data, complex geometries, and noise. This work introduces a novel, meshless approach for pressure reconstruction that leverages automatic differentiation through neural implicit representation models. Unlike conventional techniques, our method does not require grid connectivity, making it particularly effective for handling noisy, scattered, and incomplete velocity data, such as volumetric measurements from PIV and LPT. The proposed method addresses the limitations of traditional techniques like OS-MODI and GFI, which are hindered by mesh irregularities. Its meshless nature allows for seamless integration of the pressure gradient, bypassing issues with ill-conditioned meshes. Additionally, the method effectively filters out noise, ensuring robustness in noisy or incomplete datasets. By leveraging neural implicit representations, it offers a simple, cost-efficient, flexible, and scalable solution for pressure field reconstruction. This enables more accurate and reliable pressure estimates in complex fluid dynamics applications where mesh-based methods struggle.