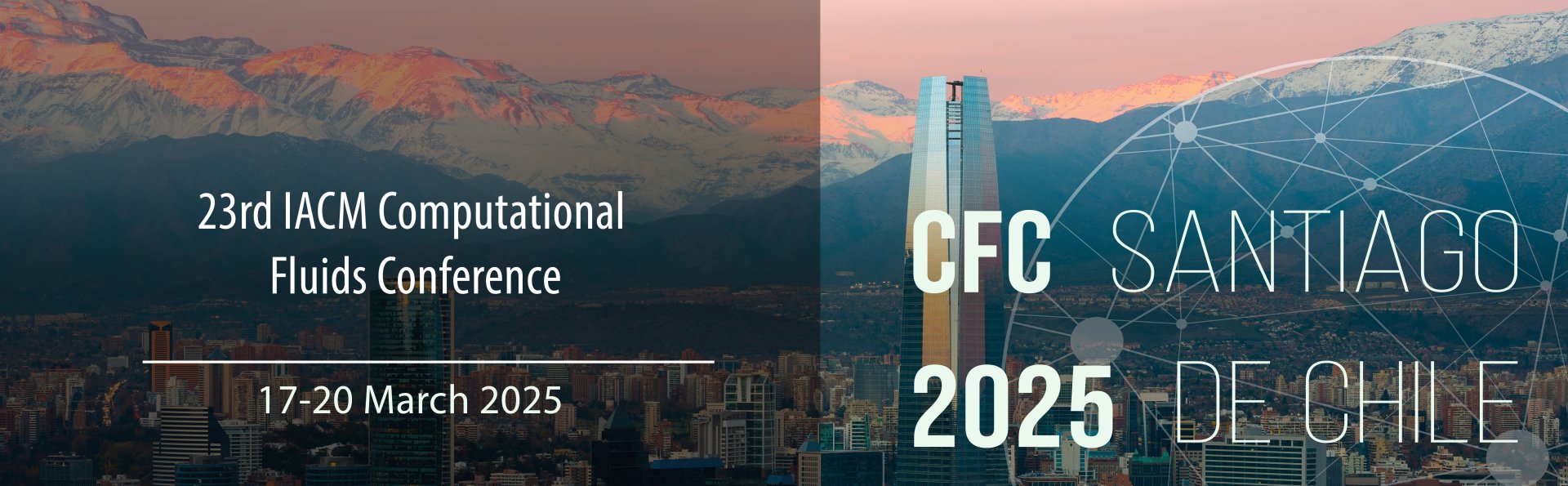
Connecting modal decompositions with instantaneously present flow structures via explainable AI
Please login to view abstract download link
In recent years, modal decompositions have become a cornerstone in computational fluid dynamics, offering powerful tools for reduced-order modeling, flow control and providing insights into de the underlying flow dynamics. However, modal representations are unable to describe flow structures that are intantaneously present in unsteady and turbulent flows. Fortunately, advances in explainable artificial intelligence (AI) have provided solutions to overcome these limitations. In particular, the Shapley additive explanations (SHAP) method is a technique that allow us to systematically quantify the impact of spatial features on model predictions, and it has recently been applied to identify regions of importance for deep learning-based predictions in wall-bounded turbulence. In this work, we develop a framework that combines SHAP with the dynamic mode decomposition (DMD) to identify regions of importance for DMD-based predictions in two distinct flow configurations: the laminar flow behind a cylinder and the turbulent wake of an urban flow. The identified regions are then leveraged to compute statistics that characterize instantaneous flow structures. We find that, for the flows studied, our combined DMD-SHAP framework identifies regions that align with the areas of maximum instantaneous turbulent kinetic energy.