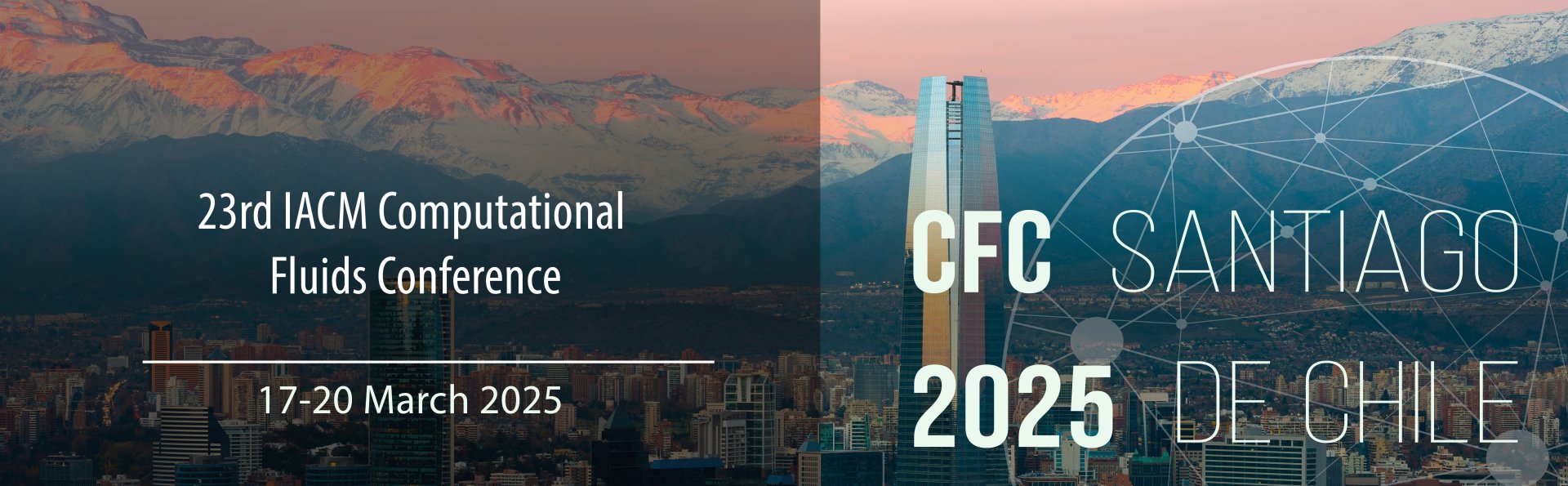
A High Reynolds Number Data-Driven Modification to the Spalart-Allmaras Turbulence Model
Please login to view abstract download link
A suite of compressible corrections has been developed for the Spalart-Allmaras turbulence model over the years. Utilizing observations from direct numerical simulations (DNS) of hypersonic turbulent boundary layers (TBL) additional modifications are proposed. First, we establish a baseline model to build upon, we elect to adapt Catris and Aupoix (SA-CA) [1] diffusion terms which satisfy the van Driest log layer solution. Our primary objective is to enhance the prediction of wall quantities in high Reynolds number hypersonic flow regimes. We propose a new near-wall damping function based on a compressible transformation of eddy viscosity, along with a modified Reynolds stress definition that accounts for Mach scaling of wall-normal Reynolds stresses. Following the approach of Spalart and Garburuk [2], who modified the destruction term to enhance low-Reynolds skin friction predictions, we aim to develop a similar modification for high Reynolds number flow (Re_theta > 6000) using data-driven methods. Specifically, we will employ symbolic regression to create a new functional expression for fw model terms, trained on extracted DNS features. Feature extraction from DNS will involve directly computing SA-CA model terms over a broad range of higher Reynolds numbers using the Mach 11 and Mach 5 flat plate boundary layer datasets provided by Haung et al. [3]. The conservative form of the SA-CA model takes the form, ... ... A DNS-approximated eddy viscosity may reveal high Reynolds number trends that are not captured in the outer flow layer. The plot above shows the component of the SA-CA equation derived from RANS and DNS simulations at an equivalent (Re_tau) locations. We plan to analyse a range of profiles across various Reynolds numbers to develop RANS-level modifications that capture any trends missed between the RANS and DNS profiles.