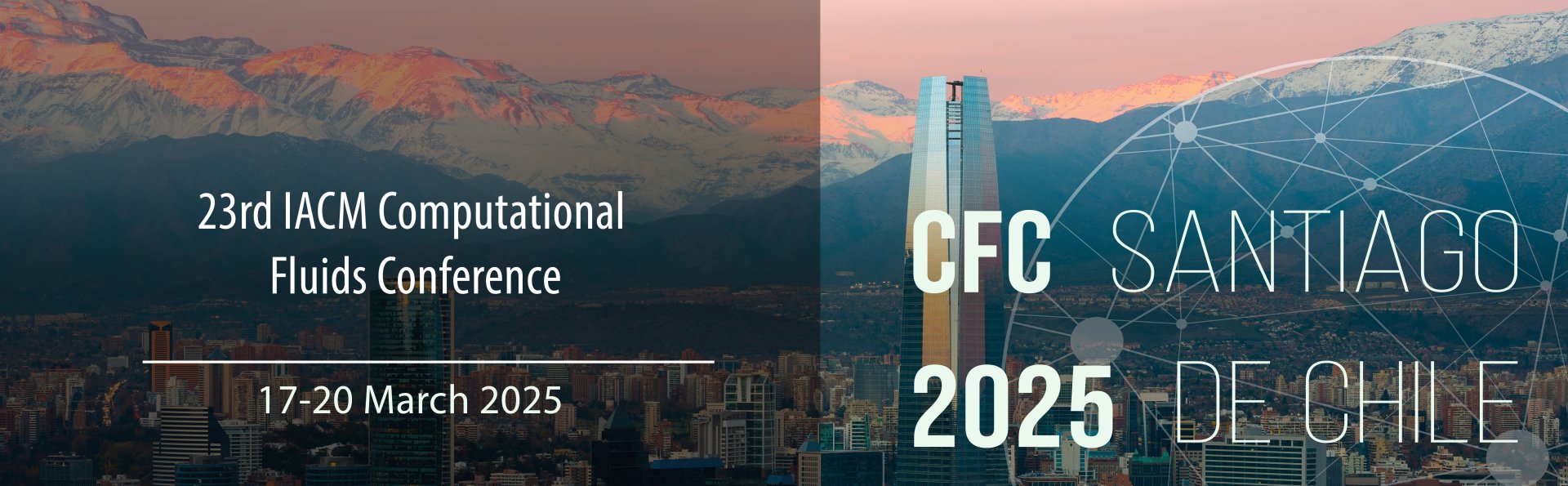
Multifidelity approaches for computational fluid dynamics in cardiovascular applications
Please login to view abstract download link
Computational fluid dynamics (CFD) plays a critical role in cardiovascular (CV) modeling, enabling precise hemodynamic assessments and improving our understanding of health conditions. However, traditional full-order models (FOMs) face significant challenges in terms of computational efficiency, particularly in real-time or many-query scenarios. To address these limitations, reduced-order modeling (ROM) and multifidelity approaches have emerged as powerful solutions, facilitating efficient simulations while retaining accuracy. This work presents two studies leveraging these methodologies. The first study focuses on multifidelity and multiphysics modeling to predict the progression and rupture risk of aortic aneurysms. Combining high-fidelity CFD simulations with graph neural networks (GNNs), we predict hemodynamic indicators such as Wall Shear Stress (WSS) and Oscillatory Shear Index (OSI) across different growth stages of abdominal aortic aneurysms. In the second application, we develop a projection-based ROM framework for unsteady parametrized optimal control problems in CV flow dynamics. Our methodology optimizes outflow boundary conditions to minimize the discrepancy between target and computed flow velocity profiles, governed by the unsteady Navier–Stokes equations with parametric dependence on the Reynolds number. Validation on vascular models, including an idealized bifurcation and a patient-specific coronary artery bypass graft, demonstrates consistent speed-up and robust performance.